As Karabulut mentioned (2022), demand forecasting is one of the biggest challenge for supply chain leaders / retail executives. As we can forecast demand, we can plan the productivity and budgeting. With lack of demand forecasting, it’s easy to become out-of-stock or overstock and increase business costs.
Weather information helps demand forecasting
Steinker et al. (2017) found that including weather information, such as sun shine hours, temperature, rain amount etc, in sales forecasting can improve the prediction performance for online stores. In Auramo(2020) and Karabulut (2022) articles, they also showed similar results that the demand forecasting with the weather adjusted data have better prediction than the baseline forecasting.
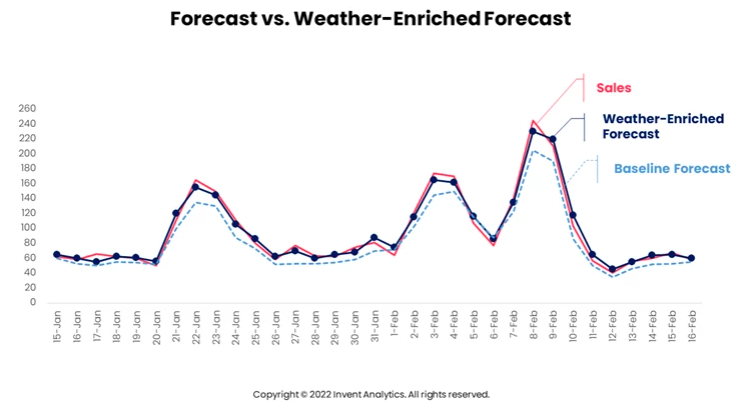
Use high resolution data
Both Bakkalbasi (2020) and Bodenstab (2016) mentioned that, in operational/supply chain planning, highly granular data is needed though aggregated weather forecasting may be more accurate. Higher granular data need higher geographical resolution to consume more information into the forecasting model. Bakkalbasi’s team called each of the granular model “micro-climate cluster”. Each of clusters has its own demand pattern, because we can include different locations and weather situations to the model to help the algorithm to specify the relationship between weather and the product demands.
Use machine learning technique
Due to include many product, location, and weather scenario information, many teams (Bodenstab (2016) and Auramo(2020)) encouraged to use machine learning technique to deal with this big amount of data. Auramo proposed that machine learning power can not only handle complicate relationship between weather scenarios and product information but also find the business decision from the analysis of weather factors.
The application of improved demand forecasting
Gold (2022) suggested that the improved forecasting can be applied in different phases: past, recent, and future. I summarized the beneficial areas as following: inventory management, marketing strategy, and pricing optimization.
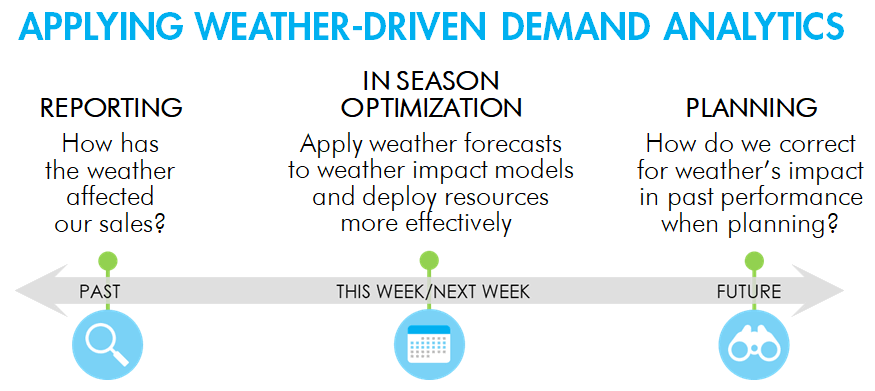
Better inventory management
With more accurate demand forecasting, we can avoid the unnecessary inventory storage rents for overstocking. For perishable products, overstocking is another extra costs should be avoided (Auramo (2020) and Gold (2022)). On the other hand, enough stock can help retail manager to understand the demand and enable to plan the productivity.
Marketing strategy
The right demand forecasting allows marketers know the categories of demands and the effectiveness of campaign, so it can help the sales the products. Gold (2022) mentioned that the forecasting helps to have better decision to know the time and region/market to use digital marketing.
Pricing optimization
Via more accurate demand forecasting, it helps us understanding the potential price options to have better revenues. Gold (2022) mentioned that the better aligned inventory can lift revenue by “higher sales, lower inventory costs, and/or by optimizing the timing and depth of markdowns”.
It’s obvious that weather situations affects people’s shopping behaviors. Thus, it’s reasonable that to include the weather information in demand forecasting may have a better performance. Based on experts suggestions, to run in operational environment, it’s better to develop the model for high geolocation resolution data and using machine learning technology. With better demand forecasting, we can save much business costs in inventory management, have effective marketing strategies, and have better pricing optimization.
References:
Auramo, V. (2022, November 3). Improve Demand Forecasting Accuracy by Factoring in Weather Impacts. Improve Demand Forecasting Accuracy by Factoring in Weather Impacts | RELEX Solutions. https://www.relexsolutions.com/resources/improve-demand-forecasting-accuracy-by-factoring-in-weather-impacts/
Bakkalbasi, O. (2020). Weather-Influenced Demand Forecasting in 2020: 6 Key Observations. Weather-Influenced Demand Forecasting in 2020: 6 Key Observations – Solvoyo. https://www.solvoyo.com/blogs/demand-forecasting/weather-influenced-demand-forecasting/
Bodestab , J. (2016, December 6). Using Weather and Climate Data to Improve Demand Forecasting. Using Weather and Climate Data to Improve Demand Forecasting | ToolsGroup. https://www.toolsgroup.com/blog/using-weather-and-climate-data-to-improve-demand-forecasting/
Gold, E. (2022, March 3). Weather Permitting: How to Use Weather Data in Retail Forecasting. Weather Permitting: How to Use Weather Data in Retail Forecasting | ToolsGroup. https://www.toolsgroup.com/blog/weather-data-retail-forecasting/
Karabulut, O. (2022, September 2). How Weather Data Improves Retail Demand Forecasting. https://www.inventanalytics.com/blog/how-weather-data-improves-retail-demand-forecasting/
Steinker, S., Hoberg, K., & Thonemann, U. W. (2017). The value of weather information for e‐commerce operations. Production and Operations Management, 26(10), 1854-1874.